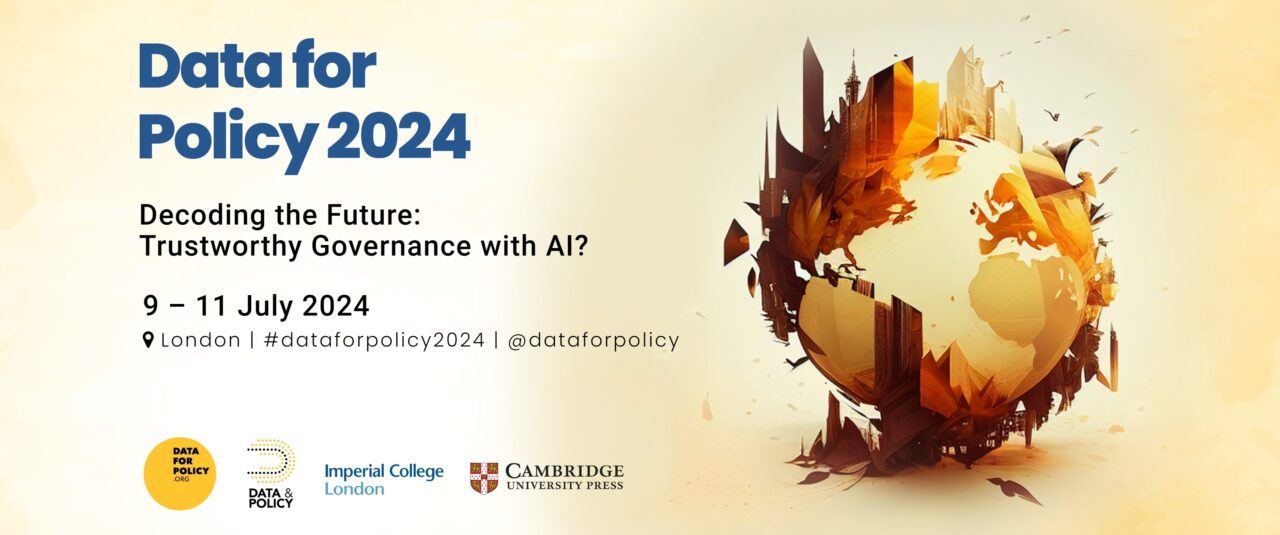
Special Track 7
Governance of Health Data for AI Innovation
Special Track Chairs:
- Renan Gadoni Canaan, University of Ottawa
- Teresa Scassa, University of Ottawa
Description
Artificial Intelligence (AI) holds immense potential in revolutionizing healthcare by leveraging comprehensive health datasets to create both public and private value. From enhanced diagnostics and drug development to personalized medicine and more efficient healthcare management, AI applications in health offer promising avenues for progress. Particularly noteworthy is its potential to detect and respond to emerging pandemics, an essential capability for regions like the Global South, where inadequate information has hindered effective responses to crises such as the Covid-19 pandemic, underscoring the importance of equitable access to healthcare. At the core of AI-driven innovation lies the indispensable role of large datasets. For AI in health, these datasets encompass a broad spectrum of health-related information, including individual health status, healthcare service delivery, resource availability, utilization, and costs. More specifically, “Clinical data” captures health information collected during patient care or formal clinical trials, while “Health Administrative data” pertains to data generated through routine healthcare services.
The rapid expansion of health data collection and analysis of individual-level datasets have brought forth pressing challenges that demand comprehensive solutions. Foremost, the sensitivity of health data puts it at risk of misuse, exploitation, or unauthorized exposure, thereby presenting tangible consequences such as denial of insurance coverage, loss of employment opportunities, or even hindrance in securing new employment. Furthermore, such unauthorized exposure may compromise individuals’ autonomy, privacy, and control over their personal information, consequently impacting their sense of dignity and self-formation.
Moreover, the digital economy has exacerbated wealth inequality, with data-driven innovations disproportionately benefiting a select few companies. While individuals often act as primary data providers, companies and institutions exploit contract and trade secret laws to de facto own the collected data. This raises concerns about fair compensation for those contributing to the data’s creation (individuals) or its curation (public institutions). Additionally, personal data can produce both positive and negative effects on other individuals within the same group. Systemic biases may lead to datasets that are incomplete, under representative or inaccurate. Lawfully collected data may be used to train algorithms and draw conclusions about individuals who did not consent to their data’s collection or use in this manner. These circumstances can lead to biased decisions that further marginalize protected groups, such as women, LGBTQI+, and indigenous communities.
The debate surrounding access to large health datasets is particularly significant in countries with universal access to public healthcare systems, where vast amounts of data are generated. Concurrently, initiatives to establish digitalized networks of data collected in such services have the potential to escalate data collection exponentially. As we traverse this intricate landscape, a profound comprehension of the governance of data becomes imperative in tackling the challenges arising from the widespread access to personal health data. Equally significant is the evaluation of how health data can be utilized to establish trustworthy governance in healthcare, thereby optimizing the benefits for both the public and private sectors in the realm of AI-driven health innovation.” Considering this, we cordially invite full-paper submissions (up to 6,000 words) to our special track from governmental organizations, academia, and the private sector addressing the following themes (inspirational rather than exhaustive):
- Data governance frameworks for accessing health data for AI innovation (clinical and/or administrative health data).
- Ethical frameworks guiding the private sector’s use of health data to create private value and drive innovation.
- Ethical considerations for governmental institutions in using health data to create public value through AI-driven initiatives.
- Addressing infrastructural, legal, and cultural challenges in providing health data access for AI innovation, especially in the Global South.
- Data governance of Health data generated by government-run and public healthcare services.